Educational Software and Machine Learning : Guide To Enhance Student Engagement
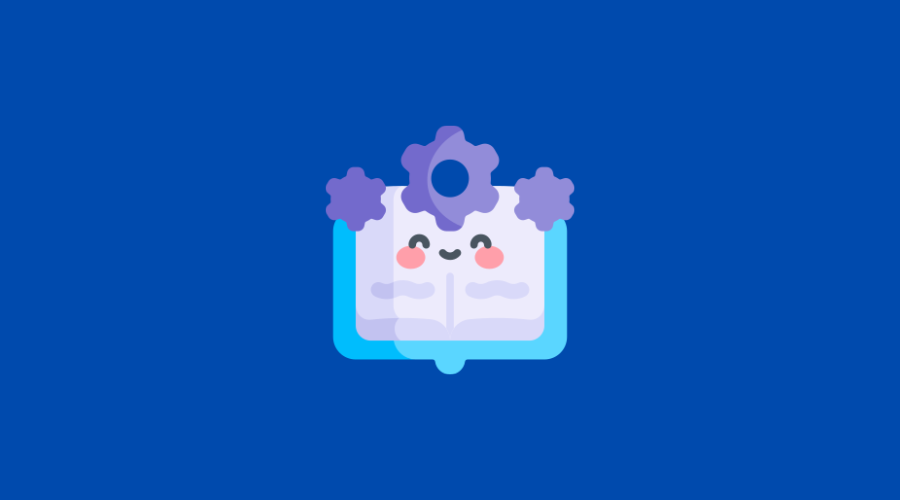
Thanks to the rise of technology, education is undergoing a big transformation. One of the most significant drivers of this change is integrating machine learning (ML) into educational software.
This technology is revolutionizing how students learn and engage with their studies, offering personalized learning experiences catering to individual needs and preferences. This article delves into how machine learning in education is leveraged to personalize educational software, enhance student engagement, and improve educational outcomes.
Without further ado, let’s get started.
The Rise of Machine Learning in Education
Machine learning, a subset of artificial intelligence, involves algorithms that learn from data and improve over time. In the context of education, ML can analyze vast amounts of data to identify patterns and make predictions. This ability is incredibly powerful for creating personalized learning experiences, as it allows software to adapt to the unique needs of each student. Platforms like Tutorhunt utilize such technology to enhance learning by connecting students with tutors that best fit their academic needs.
Personalized Learning: What Is It?
Personalized learning tailors educational content to the individual learner’s pace, preferences, and abilities. Traditional one-size-fits-all approaches often fail to address students’ diverse needs, leading to disengagement and suboptimal outcomes. Personalized learning, conversely, ensures that each student receives the right content at the right time, making learning more effective and engaging.
How Machine Learning Personalizes Education
Customized Content Delivery:
Machine learning algorithms analyze a student’s performance and learning style to adjust the difficulty and type of content delivered. The software can present more challenging material if a student excels in a particular area. Conversely, if a student struggles, it can provide additional resources and practice.
Real-Time Feedback:
Immediate feedback is crucial for effective learning. ML-powered educational software can provide instant feedback on assignments and quizzes, helping students understand and learn from their mistakes.
Predictive Analytics
Early Intervention
By analyzing student performance data, machine learning can predict which students are at risk of falling behind. Educators can intervene early, providing the necessary support to help these students catch up.
Tailored Study Plans
Predictive analytics can also help create personalized study plans that focus on areas where students need the most improvement, maximizing their learning potential.
Natural Language Processing (NLP)
Intelligent Tutoring Systems
NLP allows educational software to understand and respond to student queries in natural language. This capability makes interactions with the software more intuitive and effective, providing a more human-like tutoring experience.
Essay Grading
NLP can also be used to grade essays and provide feedback on writing assignments. This feature saves educators time and provides students with detailed, timely feedback. Educational institutions are increasingly adopting this technology through tools like college essay grader AI to ensure faster, more consistent assessment of student work
Gamification and Engagement
Adaptive Games
Educational games can be personalized using ML to match the difficulty level with the student’s ability, keeping them engaged and motivated.
Reward Systems
Machine learning can also optimize reward systems to encourage consistent effort and progress, enhancing student motivation and engagement.
Best Research and Survey Tools to Use
Benefits of Personalized Educational Software
Increased Engagement
Personalized learning experiences make education more interactive and enjoyable. They also help students engage with material tailored to their interests and abilities.
Improved Outcomes
Personalization leads to better learning outcomes by addressing the specific needs of each student. Students can progress at their own pace, ensuring they fully understand each concept before moving on.
Efficient Use of Educator Time
By automating routine tasks such as grading and content delivery, machine learning allows educators to focus on more meaningful student interactions, such as one-on-one tutoring and mentorship.
Scalability
Personalized educational software can be scaled to accommodate large numbers of students, making it a valuable tool for educational institutions of all sizes.
Real-World Applications and Success Stories
Several educational platforms have successfully integrated machine learning to enhance personalization:
Khan Academy
Khan Academy uses machine learning to personalize its educational content. The platform analyzes student performance data to recommend specific exercises and videos to help them improve in areas where they struggle.
DreamBox Learning
DreamBox Learning is an adaptive math program for K-8 students. It uses machine learning algorithms to adjust the difficulty of math problems in real time, ensuring that each student is appropriately challenged.
Coursera
Coursera, a popular online learning platform, leverages ML to recommend courses based on a user’s previous activity and learning preferences. This personalization helps learners find relevant courses that match their career goals and interests.
Challenges and Considerations
While the benefits of leveraging machine learning to personalize educational software are substantial, there are also challenges to consider:
Data Privacy and Security
Collecting and analyzing student data raises concerns about privacy and security. Educational institutions and software providers must adhere to strict data protection regulations and implement robust security measures.
Bias in Algorithms
Machine learning algorithms can sometimes reflect and perpetuate biases present in the training data. It’s essential to regularly review and update these algorithms to ensure they provide fair and unbiased recommendations.
Accessibility
Not all students have equal access to technology and the internet. Efforts must be made to ensure that personalized educational software is accessible to all students, regardless of their socioeconomic status.
The Future of Personalized Education
The future of education lies in personalization, and machine learning is the key to unlocking this potential. We can expect even more sophisticated and effective personalized learning experiences as technology advances. By embracing these innovations, educators can enhance student engagement, improve learning outcomes, and create a more equitable and effective education system.
Conclusion
Machine learning is transforming educational software by making personalized learning a reality. ML-powered educational tools enhance student engagement and outcomes through adaptive learning paths, predictive analytics, NLP, and gamification. While challenges remain, the potential benefits far outweigh the drawbacks, making machine learning an invaluable asset in the quest to provide high-quality, personalized education for every student. We can create a more inclusive, effective, and engaging learning environment by leveraging this technology.
Incorporating machine learning into educational software isn’t just a trend—it’s a revolution. The journey has just begun, and the future holds immense possibilities for learners and educators.
Comments are closed